SELF-RAG: Improving the Factual Accuracy of Large Language Models through Self-Reflection
Teaching LLMs to retrieve, generate, and critique through self-reflection
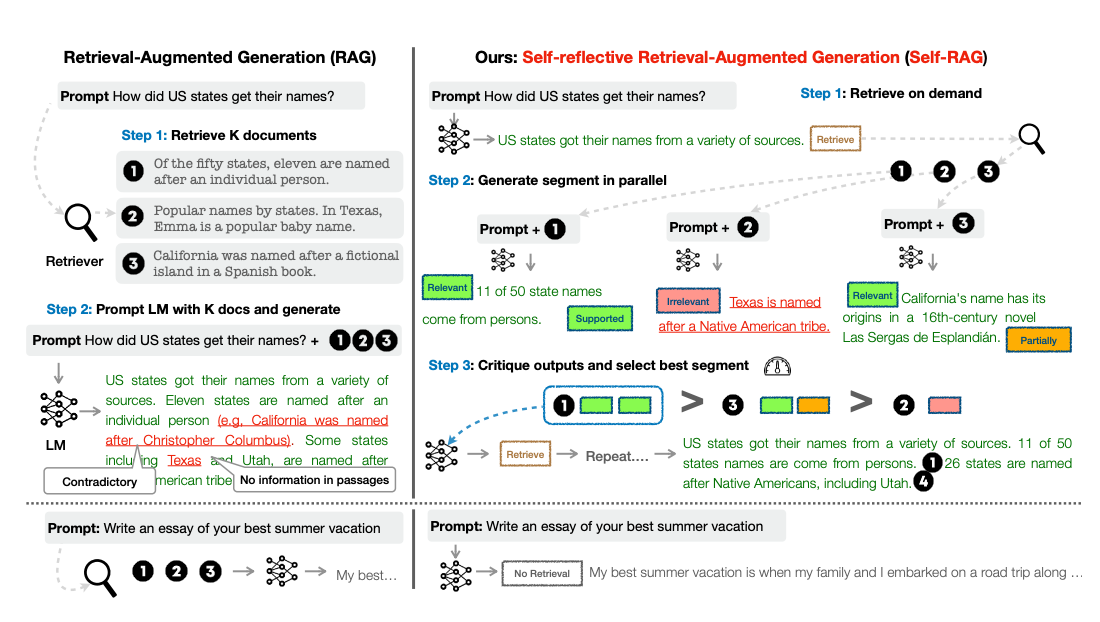
In recent years, artificial intelligence researchers have made astounding progress developing large language models that can generate remarkably human-like text. Models like GPT-3 and PaLM with billions or trillions of parameters display impressive versatility in language tasks. However, a major limitation persists - these models still frequently generate factual inaccuracies and unsupported claims. Overcoming this limitation is critical for reliably deploying LLMs in real-world applications like search engines, chatbots, and content creation tools.
A team from the University of Washington and IBM Research recently made important headway on this challenge. In a paper published on arXiv, they introduced a novel technique called Self-Reflective Retrieval-Augmented Generation (SELF-RAG) that trains LLMs to enhance their own factual accuracy through selective retrieval of knowledge and self-critiquing of generations.
The Persistent Problem of Factual Inaccuracy
Despite their human-like capabilities, LLMs inherently lack true understanding of the world. They are pattern recognition systems trained on vast amounts of text data. As a result, the knowledge encoded in their parameters is imperfect and prone to hallucination or contradiction. Prior work has shown LLMs often generate logical fallacies, unsafe advice, racist or biased statements, and other factual inaccuracies.
Keep reading with a 7-day free trial
Subscribe to AIModels.fyi to keep reading this post and get 7 days of free access to the full post archives.