Meta figured out how to make better videos by turning text into images first
The new approach uses "explicit image conditioning" for higher quality video
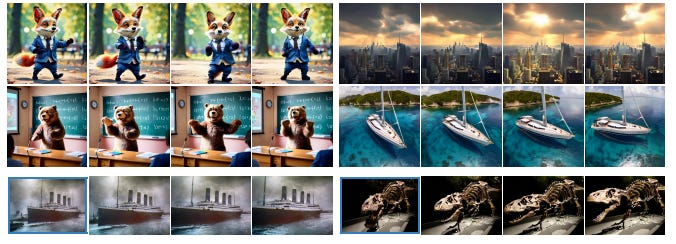
Generating diverse, convincing videos from text prompts alone remains an exceptionally challenging open problem in artificial intelligence research. Directly translating text descriptions into realistic, temporally coherent video sequences requires modeling intricate spatiotemporal dynamics that continue to confound current systems.
In a new paper, researchers from Meta introduce an innovative text-to-video generation approach called Emu Video that they claim sets a new state-of-the-art standard. By factorizing the problem into explicit image generation followed by video generation conditioned on both text and image, the authors claim Emu Video produces substantially higher quality and more controllable results. Let's see what they built and how their approach holds up.
The Text-to-Video Challenge
Applications like automated visual content creation, conversational assistants, and bringing language to life as video require automatic text-to-video generation systems. However, modeling the complex world dynamics and physics required for plausible video synthesis poses a formidable challenge for AI.
Videos demand exponentially more complex scene understanding and reasoning compared to static images. Beyond just recognizing objects depicted in the text prompt, generating coherent video requires inferring how objects interact, move, deform, occlusion relationships, and other changes over time-based on the textual description.
This requires the model to have a strong grasp of real-world semantics, cause and effect, object permanence, and temporal context to produce videos that align with human sensibilities.
Directly predicting a sequence of video frames from only text input is exceptionally difficult due to the high dimensionality and multi-modality of the video space. Without sufficiently strong conditioning signals to constrain the output, existing text-to-video systems struggle to generate consistent, realistic results at high resolution (e.g. 1024x1024 pixels) that faithfully reflect the input textual descriptions.
They often produce blurry outputs that lack fine details, exhibit temporal jittering or instability across frames, fail to fully capture the semantics of the text prompt, and collapse to mean, average outputs that do not match the specificity of the description.
Emu Video: Factorized Text-to-Video Generation
To address these challenges, the key insight behind Emu Video is to factorize the problem into two steps:
Keep reading with a 7-day free trial
Subscribe to AIModels.fyi to keep reading this post and get 7 days of free access to the full post archives.