Combining Thermodynamics and Diffusion Models for Collision-Free Robot Motion Planning
A new AI-based method to help robots navigate 3D environments
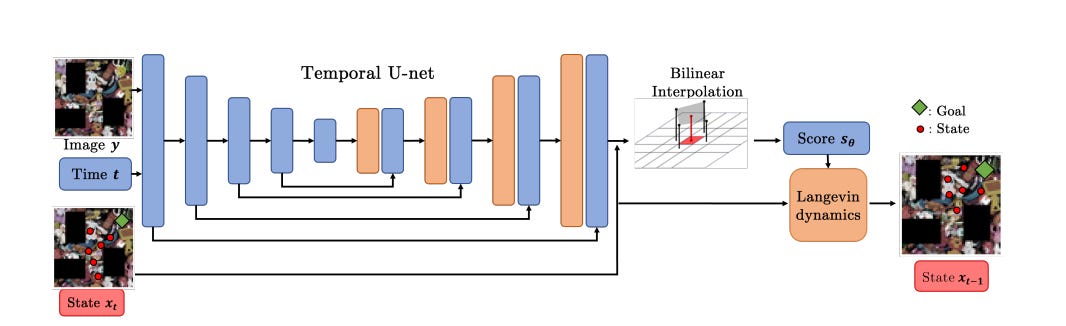
Researchers from Yonsei University and UC Berkeley recently published a paper describing an innovative artificial intelligence technique for improving robot navigation in complex, unpredictable environments. Their method leverages a tailored diffusion model to generate collision-free motion plans from visual inputs. This approach aims to address long-standing challenges in deploying autonomous robots in unstructured real-world spaces. Let's see what they found, and assess how generalizable these findings will be to fields like warehouse robotics, manufacturing, and self-driving cars.
The Persistent Obstacles to Practical Robot Navigation
Enabling nimble and reliable robot navigation in unconstrained spaces remains an elusive grand challenge in the field of robotics. Robots hold immense potential to take on dangerous, dull, dirty, and inaccessible tasks in domains like warehouses, mines, oil rigs, surveillance, emergency response, and ... elder care. However, for autonomous navigation to become practical and scalable, robots must competently handle changing environments cluttered with static and dynamic obstacles.
Several stubborn challenges have impeded progress:
Mapping Requirements - Most navigation solutions demand an accurate 2D or 3D map of the environment beforehand. This largely constrains robots to narrow industrial settings with fixed infrastructure. Pre-mapping and localization are infeasible in highly dynamic spaces.
Brittleness to Unexpected Obstacles - Robots struggle to respond in real-time to novel obstacles and changes in mapped environments. Even small deviations can lead to catastrophic failures.
Keep reading with a 7-day free trial
Subscribe to AIModels.fyi to keep reading this post and get 7 days of free access to the full post archives.